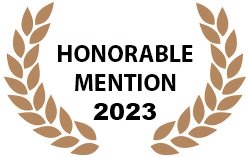
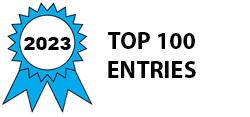
When we work with others in teams, we implicitly infer each other's intentions, beliefs, and desires and use this information to coordinate our actions. We believe that the same should hold for collaborative robots if they are to perform efficiently as part of human-robot teams. Robots should understand how we intend to do a specific task, such as furniture assembly, anticipate our next actions, and proactively support us by delivering the right parts or tools.
A widely used technique for robot training is learning from demonstration, where human experts provide multiple demonstrations of how they prefer to perform a given task. However, many tasks that would benefit from robotic assistance are long and tedious. For instance, imagine having to assemble an IKEA bookcase ten times just to show the robot how you would like to perform the assembly.
However, in this new study, we have found substantial similarities in how individuals may assemble different products. For example, if a user picks up the heaviest part of the assembly first, they are likely to do the same in a completely different assembly. There are factors related to physical and mental effort that are not specific to a particular assembly but influence how people prioritize their actions.
Therefore, instead of having users provide demonstrations on the actual product, we asked them to assemble a much shorter task that they could quickly and easily perform. We then developed an AI algorithm that utilized the user demonstrations on the canonical task to predict their actions in the actual task.
This enabled the robot to proactively support users in a longer assembly by delivering parts and tools they would need in advance, even without having observed any of these users perform the assembly before! As a result, there was a significant efficiency gain, with demonstrations on the short task being 2.3 times faster compared to demonstrations on the actual, longer assembly.
This work has the potential to bring about dramatic improvements in human productivity by shaping how collaborative robots anticipate our actions and support us in performing tedious tasks both in the workplace and at home. Robots can support people by performing tasks that are unsafe or dangerous, for instance carrying a heavy part or picking up parts that would require the worker to go into ergonomically unsafe postures.
Our contribution is an AI algorithm that is not tied to specific robot hardware, allowing for a wide range of applications, from aerospace manufacturing and electronics assembly to home maintenance and household chores, saving time and money and improving quality of life.
For more information, see our paper that was a Best Systems Paper finalist in the 2023 ACM/IEEE International Conference of Human-Robot Interaction:
https://dl.acm.org/doi/10.1145/3568162.3576965
News article on our research: https://viterbischool.usc.edu/news/2023/04/robots-predict-human-intention-for-faster-builds/
Video
-
Awards
-
2023 MRobotics & Automation Honorable Mention
-
2023 Top 100 Entries
Voting
-
ABOUT THE ENTRANT
- Name:Caitlin Dawson
- Type of entry:teamTeam members:
- Heramb Nemlekar
- Caitlin Dawson
- Software used for this entry:https://youtu.be/GmAVxtqWDxM
- Patent status:none