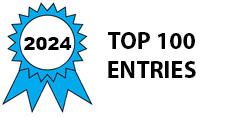
First-person view (FPV) drone racing is a televised sport where professional competitors pilot high-speed aircraft through a three-dimensional circuit, achieving astonishing speeds. We built the first AI pilot that flies faster than human world champions: Swift.
This breakthrough represents a significant step forward in robotics research. Swift's ability to achieve champion-level performance in racing could unlock new possibilities for autonomous aircraft operating in challenging environments. Furthermore, it could pave the way for the next generation of robots that can perform tasks better than human operators. Examples of such tasks include inspection and mapping missions in industrial environments, search and rescue flights, or public safety and monitoring. All those applications would benefit from being performed faster, cheaper, and more autonomously. Our achievements have been published in the famous research journal Nature and covered by the press in premier research outlets, including IEEE Spectrum and Forbes.
Swift is trained via deep reinforcement learning to race small drones through complex race tracks. Swift's ability to race competitively was tested against three human world champions on a professionally designed track. Despite the pilots having over one week of practice time, Swift won multiple races, demonstrating the fastest recorded race time. This was accomplished with identical drones regarding weight, shape, and propulsion, showcasing that Swift's performance wasn't attributed to hardware advantages but to its learning-based control.
We use model-free, on-policy deep reinforcement learning to train our system in a simulated environment to develop its control policy. Non-parametric empirical noise models, estimated from real-world data, were integrated to account for discrepancies in sensing and dynamics between the simulated and physical environments. This enables Swift to surpass the racing speeds achieved by previous autonomous systems that depended on external motion-tracking hardware, even while solely relying on its onboard sensing capabilities, namely the camera and inertial measurement unit.
Video
-
Awards
-
2024 Top 100 Entries
Voting
-
ABOUT THE ENTRANT
- Name:Elia Kaufmann
- Type of entry:individual
- Patent status:none